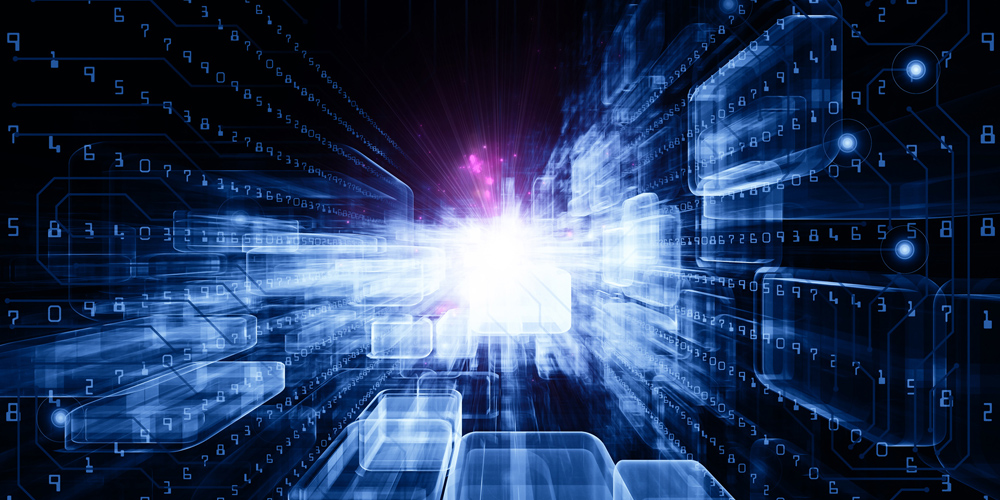
ARL intelligent systems S&T is leading to a tactical offset strategy for operations in a range of challenging settings.
by Dr. Brian M. Sadler
The desire for a third offset strategy has been a major focus of DOD science and technology (S&T) discussion for the past few years. While the Navy and Air Force primarily face issues related to large-scale, extended-range operations, the Army must address a different set of long-term challenges in complex operational scenarios. These notably include megacities and other perplexing domains such as subterranean and jungle environments. It is not difficult to envision a megacity in a developing nation facing a quicksand of natural disaster, failing infrastructure, tribal conflict and fast-spreading disease, and the subsequent call for Army operations. Other potential scenarios may prove equally challenging, complex and risky. These expeditionary Army operations likely will entail high-risk and slow operational tempo (OPTEMPO), be manpower intensive and require difficult large-scale logistic deployment.
The application of man-unmanned machine teaming (MUM-T) and autonomous systems is a potential offset strategy. Many recent studies and workshops (e.g., the Defense Science Board, the National Academies, the Defense Advanced Research Projects Agency, the Army Science Board and the U.S. Army Research Laboratory (ARL)) have reinforced the operational and technological potential of MUM-T. It is clear that if successfully developed, autonomy offers leap-ahead capability and the potential for a third offset in sea and air operations. Distributed collaborative autonomous systems, teamed with Soldiers, offers a tactical offset strategy; a means to operate in complex urban and other domains at high tempo, with significantly reduced risk, and with fewer Soldiers.
Army application of collaborative intelligence
At ARL, our tactical offset vision is to develop the underpinning S&T for highly distributed and collaborative intelligent systems, consisting of air and ground robotic and manned platforms, high-performance tactical computing, knowledge bases and sensors, all connected to local and remote Soldiers via a self-healing network. The heterogeneous, interconnected mix of large and small platforms provides a rich potential to exploit autonomy for situational awareness, protection and networking. Examples include using autonomy for rapid intelligence, surveillance and reconnaissance ahead of and around dismounts, emplacing fixed or mobile sensor networks, providing a bubble of protection around moving Soldiers, and deploying swarms of small autonomous aerial vehicles moving at up to ballistic rates.

TEAM BUILDING
Teaming Soldiers with distributed collaborative autonomous systems offers a means to operate in complex urban and other domains at high tempo and with significantly reduced risk. (ARL Public Affairs)
ARL’s goal in developing the S&T behind intelligent systems is to enable man-machine teaming to provide tactical offset by:
- Providing large networks of heterogeneous intelligent agents that can coordinate and rapidly distribute themselves based on commander’s intent.
- Extending reach and vision into large, complex environments beyond the limits of national assets.
- Collaboratively perceiving the environment and providing situational awareness against dynamic threats.
- Analyzing information to enable rapid human/intelligent system decision-making and adaptable mission profiles.
- Strategically assessing risk and directing intelligent and efficient use of force against dynamic threats.
Axes of complexity
Several key factors dictate the difficulty of the Army problem and limit the operational capability of any given state-of-the-art autonomy technology suite:
- Complexity of the operational environment. Megacities are an extreme example of a complex environment. It is far easier to navigate an autonomous vehicle in open air or water than into buildings or tunnels.
- Available infrastructure. Prior knowledge of the environment, massive networking and power sources are generally available for commercial applications, but not in Army scenarios.
- Operational tempo. Artificial intelligence algorithms are often so complex they are beyond our current ability to implement them for real-time operation in small platforms that cannot rely on extensive infrastructure.
There is also the complexity of system design, which increases with the number of agents; the variety of agent types; agent complexity and adaptability; and the degree of interaction and communication needed among the agents (for both machine and human interaction). Tactical application is reliant on heterogeneous architectures across Army platforms, networks, sensors and processors, which also raises questions of logistics and sustainability. However, we currently do not have a full understanding of how to design a system consisting of hardware and software modules that can be composed and assembled, and that map to efficient hardware.

WHAT WILL THE NEXT MISSION LOOK LIKE?
The Army faces long-term challenges in complex operational scenarios. A system that works in an urban environment might not be adaptable to a megacity, jungles, caves or a subterranean environment. (ARL Public Affairs)
Autonomous networking
The tactical offset strategy inherently relies on communications among autonomous nodes, sensors, knowledge bases and humans. Recent ARL research has definitively shown that viewing networking as a separate add-on component leads to distinctly suboptimal results. For example, when autonomous agents collaborate to explore and map a building, the task can be much more efficiently accomplished when the agents account for radio connectivity and plan for information sharing and exchange.
The design of collaborative intelligent systems to meet the tactical offset vision must include networking as an integrated component. This will take advantage of large air and ground platforms with less restrictive power and computation requirements, and exploit cognitive radio techniques to efficiently manage spectrum usage and network capacity. The resulting system will be resilient, using autonomous nodes to self-heal the network, reconfiguring depending on the task, and adapting to threats.
Advances in autonomy and artificial intelligence
Implementing the tactical offset vision hinges on successful ongoing research and development that seeks to accelerate and merge advances in cognition and artificial intelligence (AI) with advances in collaborative robotics and cognitive networking. Technology convergence continues to unite networking, processing, sensing and control onto small, mobile air and ground platforms. This follows large commercial investment and mass production trends in cellular, robotic and sensor technologies.

INTELLIGENT SYSTEM COMPONENTS
A tactical offset strategy—a means to operate in complex urban and other domains at high tempo, with significantly reduced risk and with fewer Soldiers—relies on communications among autonomous nodes, sensors, knowledge bases and humans. (ARL Public Affairs)
With a decade of Army basic research 6.1 investment in programs such as the ARL Micro Autonomous Systems and Technology Collaborative Technology Alliance, new small-scale mechanical platforms (e.g., quadrotors) are now a commercial commodity, and algorithms for autonomous control, sensing, navigation and mapping have been demonstrated.
Artificial neural networks (ANNs) have exploded into a variety of commercial applications since 2010. While the basic ANN technology dates to the 1990s, two trends have enabled their recent emergence. First, digital processing technology has continued its rapid advancement as predicted by Moore’s Law, such that these algorithms from the 90’s can now be computed in reasonable time on laptop quality processors. Second, it is now technically feasible to collect and use training data sets at the very large scale needed to ensure good statistical performance with brute-force learning algorithms. Through trial and error, it became apparent that the best performance could require millions of training examples. While it is time consuming to collect such large validated data sets, digital hardware advances have made it possible to use them to train ANNs.
Embraced by large U.S. commercial enterprises such as Google and Facebook, ANNs have been successfully applied in such areas as image processing and vision, natural language processing, robotics and multi-agent systems. They have displaced decades-old technologies in image and speech processing. ANNs are now better than humans on some kinds of visual object and word recognition, not to mention gaming. The use of ANNs has enabled driverless cars, whose development is limited only by cost, legal regulation and reliance on fixed infrastructure such as maps, roadside electronic aids, signs, road markings and networking.
ANNs typically process one input and one output at a time, such as detecting objects in an image. An ANN variant called a recursive neural network (RNN), also dating to the 1990s, enables sequence processing, such as entire spoken sentences or a sequence of video frames. Other ANN variants combine the above to build a system that allows a user to verbally ask a question about an image, for example, with the system responding verbally with an answer.

CONNECTING THE DOTS
Rapid querying and information retrieval have been made possible by parallel advances in mining big data to produce knowledge bases and systematic methods for the storage, assimilation and association of data. (Shutterstock image by agsandrew)
Parallel advances in mining big data to produce knowledge bases has produced systematic methods for the storage, assimilation and association of data, enabling rapid querying and information retrieval. Knowledge bases form the memory of intelligent systems like IBM’s Watson (famous for its performance against human experts in the TV game Jeopardy), computer game-playing architectures and numerous other applications. Machine learning can be thought of in this vein, exploiting data to learn and specify models that can make predictions.
Conclusion
ANN, knowledge base and machine learning technologies have advanced rapidly in this decade. Combining these with recent progress in robotics and autonomy, the Army is poised to develop the underpinning S&T for highly distributed and collaborative intelligent systems that can provide a tactical offset strategy for envisioned operations in megacities and other challenging scenarios.
For more information, go to http://www.arl.army.mil/www/default.cfm?page=2637.
DR. BRIAN M. SADLER is the Army senior scientist for intelligent systems at ARL. He has a Ph.D. from the University of Virginia, and an M.S. and B.S. from the University of Maryland, all in electrical engineering. He is a Fellow of the Institute of Electrical and Electronics Engineers and ARL.
This article was originally published in the April – June issue Army AL&T magazine.
Subscribe to Army AL&T News, the premier online news source for the Acquisition, Logistics, and Technology (AL&T) Workforce.
ONLINE EXTRAS
- “A Review of 40 Years of Cognitive Architecture Research: Focus on Perception, Attention, Learning and Applications,” October 2016
- “Research and vision for intelligent systems for 2025 and beyond,” Small Wars Journal, April 2016.
- “Deep Learning,” MIT Press Book, 2016
- “Center for Strategic and Budgetary Assessments,” by Robert Martinage, October 2014
- “Megacities and the United States Army: Preparing for a Complex and Uncertain Future,” Chief of Staff of the Army, Strategic Studies Group, June 2014