
Rosie the robot learns about the world, with help from a plethora of ARL-designed sensors
by Dr. Joseph Conroy and Mr. Earl Jared Shamwell
If you ever watched “The Jetsons,” an animated sitcom (1963-1964) about a family living in fictional Orbit City in the 2060s, you likely remember the iconic depiction of a futuristic utopia complete with flying cars and robotic contraptions to take care of many human needs. Robots, such as sass-talking housekeeper Rosie, could move through that world and perform tasks ranging from the mundane to the highly complex, all with human-like ease.
In the real world, however, robotic technology has not matured so swiftly.
What will it take to endow current robots with these futuristic capabilities? One place to look for inspiration is in human behavior and development. From birth, each of us has been performing a variety of tasks over and over and getting better each time. Intuitively, we know that practice, practice and more practice is the only way to become better at something. We often say we are developing a “muscle memory” of the task, and this is correct in many ways. Indeed, we are slowly developing a model of how the world operates and how we must move to influence the world. When we are good at a task—that is, when our mental model well captures what actually happens—we say the task has become second nature.
‘WHAT A PIECE OF WORK IS A MAN’
Let’s consider for a moment several amazing tasks performed by humans just for recreational purposes. Baseball players catch, throw and hit a ball that can be moving faster than 100 miles per hour, using an elegant fusion of visual perception, tactile sensing and motor control. Responding to a small target at this speed requires that the muscles react, at least to some degree, before the conscious mind fully processes visually what has happened.
The most skilled players of the game typically have the best mental models of how to pitch, hit and catch. A mental model in this case contains all the prior knowledge and experience a player has about how to move his or her body to play the game, particularly for the position. The execution of an assumed mental model is called “feed forward control.” A mental model that is incorrect or incomplete, such as one used by an inexperienced player, will reduce accuracy and repeatability and require more time to complete a task.

WIRED FOR DISCOVERY
Earl Shamwell, one of the authors, sets up a multisensor robotics testbed to collect images, LIDAR data and inertial measurements. Researchers aim to improve robotic performance by closing the gap between what a robot expects to happen and what actually happens. (Photo by Jhi Scott, ARL)
We can assume that even professional baseball players would need significant time to adjust if they were magically transported to play on the moon, where gravity is much weaker and air resistance is nonexistent. Similarly, another instance of incorrect models can be observed in the clumsy and uncoordinated movements of quickly growing children; their mental models of how to relate to the world must constantly change and adapt because they are changing. Nevertheless, humans are quite resilient to change and, with practice, they can adapt to perform well in new situations.
A major focus of much current research going on now at the U.S. Army Research Laboratory (ARL) is moving toward creating a robot like Rosie, capable of learning and executing tasks with the best precision and speed possible, given what we know about our own abilities.
NOT QUITE ‘INFINITE IN FACULTY’
In general, we can say that Rosie-like robot performance is possible given sufficient advances in the areas of sensing, modeling self-motion and modeling interactions with the world.
Robots “perceive” the world around them using myriad integrated sensors. These sensors include laser range scanners and acoustic ranging, which provide the distance from the robot to obstacles; cameras that permit the robot to see the world, similar to our own eyes; inertial measurement sensing that includes rate gyroscopes, which sense the rate of change of the orientation of the robotic device; and accelerometers, which sense acceleration and gravity, giving the robot an “inner ear” of sorts. All these methods of sensing the world provide different types of information about the robot’s motion or location in the environment.
Sensor information is provided to the algorithms responsible for estimating self-motion and interaction with the world. Robots can be programmed with their own versions of mental models, complete with mechanisms for learning and adaptation that help encode knowledge about themselves and the environment in which they operate. Rather than “mental models,” we call these “world models.”
‘IN FORM AND MOVING HOW EXPRESS AND ADMIRABLE,’ SORT OF
Consider a robot acting while assuming a model of its own motion in the world. If the behavior the robot actually experiences deviates significantly from the behavior the robot expects, the discrepancy will lead to poor performance: a “wobbly” robot that is slow and confused, not unlike a human after too many alcoholic beverages. If the actual motion is closer to the anticipated model, the robot can be very quick and accurate with less burden on the sensing aspect to correct for erroneous modeling.
Of course, the environment itself greatly affects how the robot moves through the world. While gravity can fortunately be assumed constant on Earth, other conditions can change how a robot might interact with the environment. For instance, a robot traveling through mud would have a much different experience than one moving on asphalt. The best modeling would be designed to change depending on the environment. We know there are many models to be learned and applied, and the real issue is knowing which model to apply for a given situation.
Robotics today are developed in laboratory environments with little exposure to the variability of the world outside the lab, which can cause a robot’s ability to perceive and react to fail in the unstructured outdoors. Limited environmental exposure during model learning and subsequent poor adaptation or performance is said to be the result of “over-fitting,” or using a model created from a small subset of experiences to maneuver according to a much broader set of experiences.
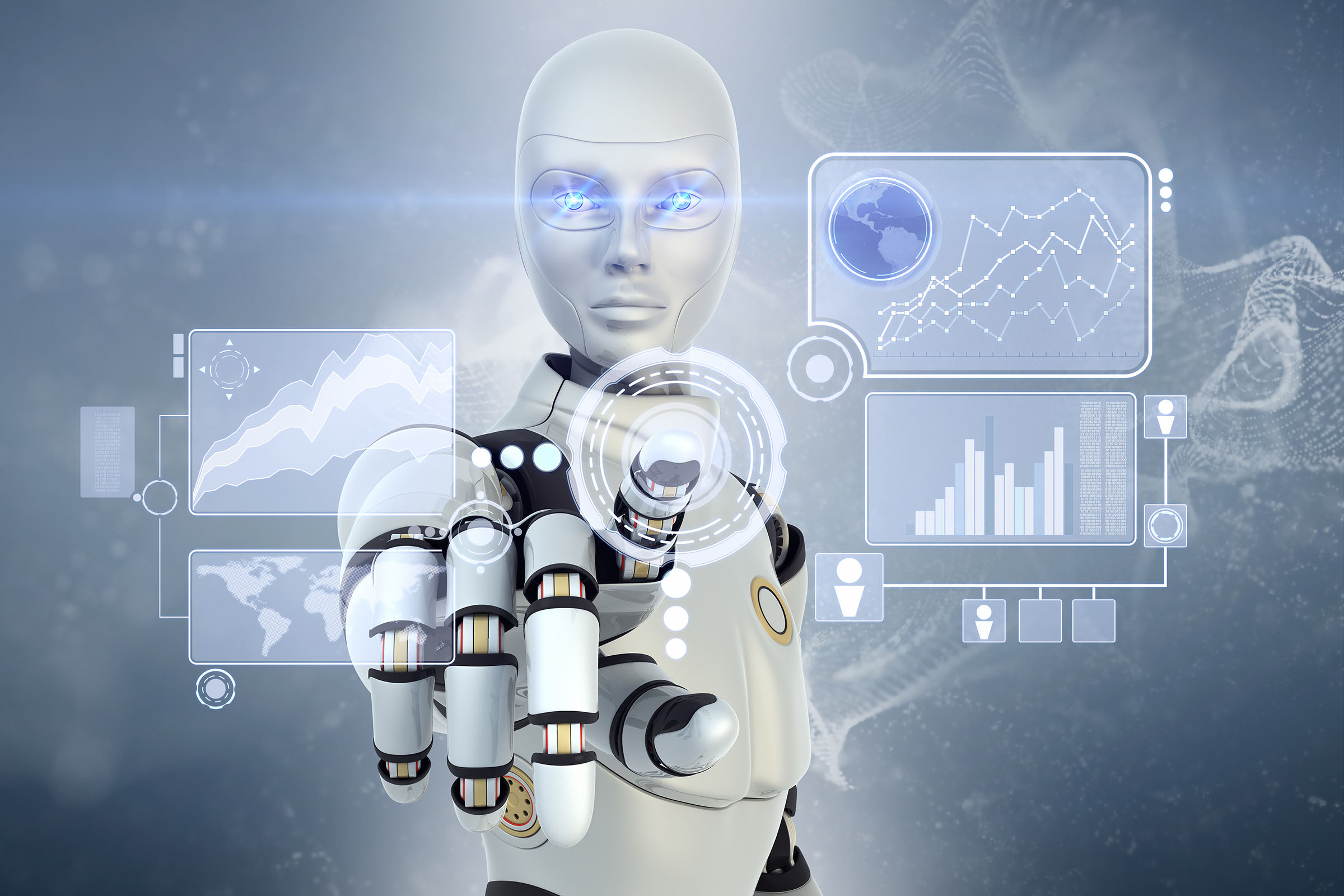
Researchers at ARL are exploring methods for robots to learn and use models that enable faster autonomy by assessing when and under what conditions different methods of sensing perform well or poorly. (Image by iLexx/iStock)
CONCLUSION
At ARL, we are researching specific advances to address these areas of sensing, modeling self-motion and modeling robotic interaction with the world, with the understanding that doing so will enable great enhancements in the operational speed of autonomous vehicles.
Specifically, we are working on knowing when and under what conditions different methods of sensing work well or may not work well. Given this knowledge, we can balance how these sensors are combined to aid the robot’s motion estimation.
A much faster estimate is available as well through development of techniques to automatically estimate accurate models of the world and of robot self-motion. With the learned and applied models, the robot can act and plan on a much quicker timescale than what might be possible with only direct sensor measurements.
Finally, we know that these models of motion should change depending on which of the many diverse environmental conditions the robot finds itself in. To further enhance robot reliability in a more general sense, we are working on how to best model the world such that a collection of knowledge can be leveraged to help select an appropriate model of robot motion for the current conditions.
If we can master these capabilities, then Rosie can be ready for operation, lacking only her signature attitude.
For more information about ARL collaboration opportunities in the science for maneuver, go to http://www.arl.army.mil/opencampus/.
DR. JOSEPH CONROY is an electronics engineer in ARL’s Micro and Nano Materials and Devices Branch. He holds a doctorate, an M.S. and a B.S., all in aerospace engineering and all from the University of Maryland, College Park.
MR. EARL JARED SHAMWELL is a systems engineer with General Technical Services LLC, providing contract support to ARL’s Micro and Nano Materials and Devices Branch. He is working on his doctorate in neuroscience from the University of Maryland, College Park, and holds a B.A. in economics and philosophy from Columbia University.
This article will be published in the January – March 2017 issue of Army AL&T Magazine.
Subscribe to Army AL&T News, the premier online news source for the Acquisition, Logistics, and Technology (AL&T) Workforce.