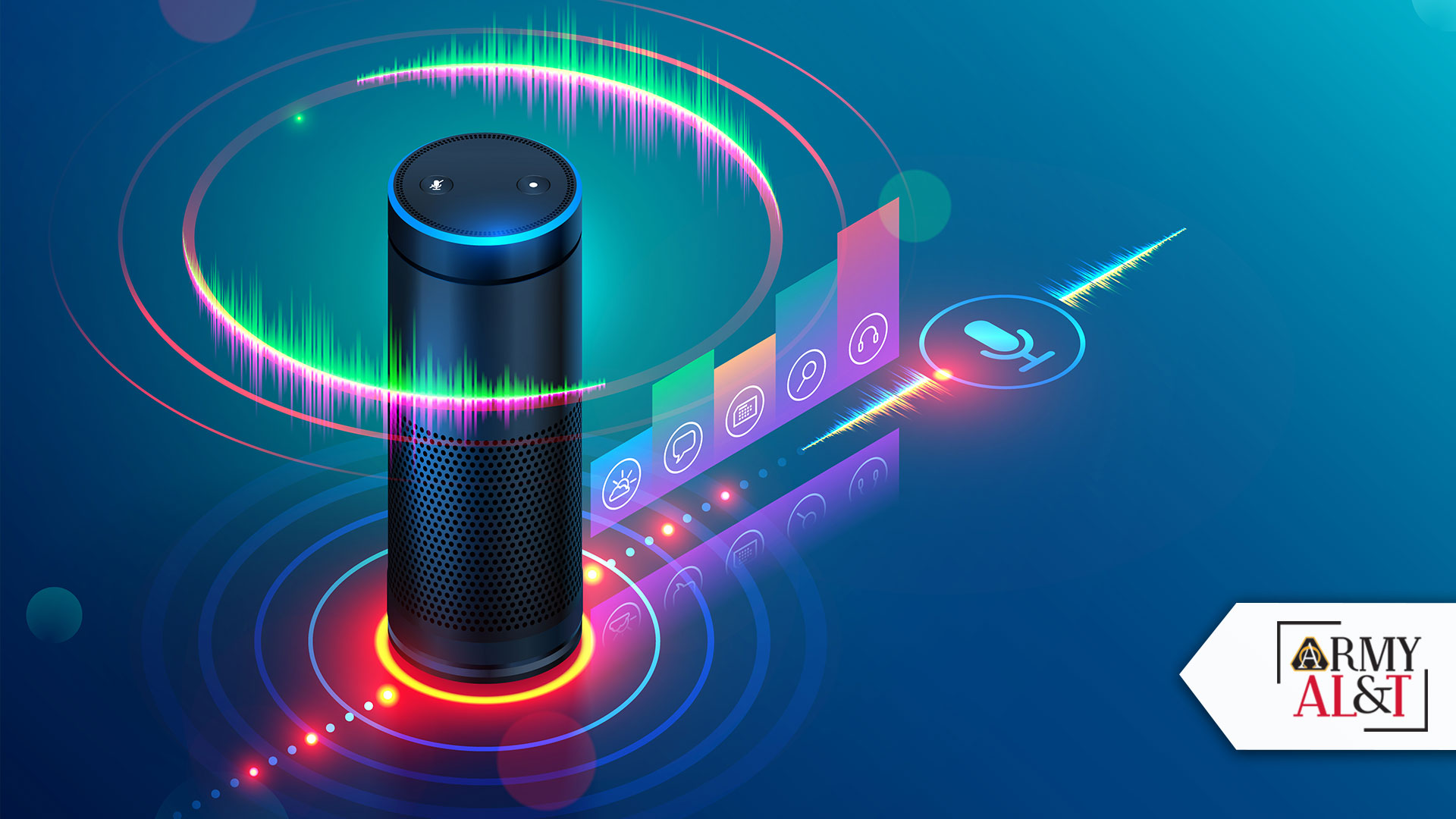
LET ME REPEAT: Current AI agents consume each request independently of the user’s history—it’s like having a conversation with a goldfish. (Image by Getty Images)
Tactical speech recognition presents a variety of challenges that the Army is looking to overcome.
by Thom Hawkins and Dr. Reginald Hobbs
“Agent, provide data about that base.”
“I found ‘All About that Bass’ by Meghan Trainor. Do you want me to play it?”
“Agent, no! Data about that base!”
Because you know I’m all about that bass, ‘bout that bass. No treble …
This is a nightmare scenario for our Soldiers, but also sounds like a plausible dialogue given our collective experience with commercial virtual assistants like Amazon’s Alexa.
There’s a reason that we put up with the flaws—because we’ve been raised with high expectations for this type of interface. Voice interaction with artificial intelligence has a long history in Hollywood—from Robby the Robot of “Forbidden Planet” (1956) and HAL 9000 from “2001: A Space Odyssey” (1968) through Tony Stark’s JARVIS in “Iron Man” (2008) and Ava from “Ex Machina” (2014)—because the technology enables human-like interaction to further the film’s narrative without boring anyone with typing.. Because of this familiarity bred through popular science fiction, we expect these voice interfaces to work, regardless of the environment and context.
Apple’s Siri, one of the first widely available virtual assistants, was introduced on the iPhone 4S in 2011. Many of our Soldiers have never owned a mobile phone that did not include such an agent. One of the biggest challenges we have to technology adoption is managing the expectations of users who are exposed not only to military technology, but also commercial. A user might be frustrated when an action on a hand-held device takes several more steps than it would on their own mobile phone.
The use cases for automatic speech recognition in a tactical environment include voice control, conversational artificial intelligence (AI) agents and content analysis of voice communication, all of which have roots in available commercial technology. Though voice recognition and speech recognition are sometimes used synonymously, there is an important distinction between the two—voice recognition identifies people by the sound of their voices, while speech recognition identifies people by the content (i.e., words) of their speech.
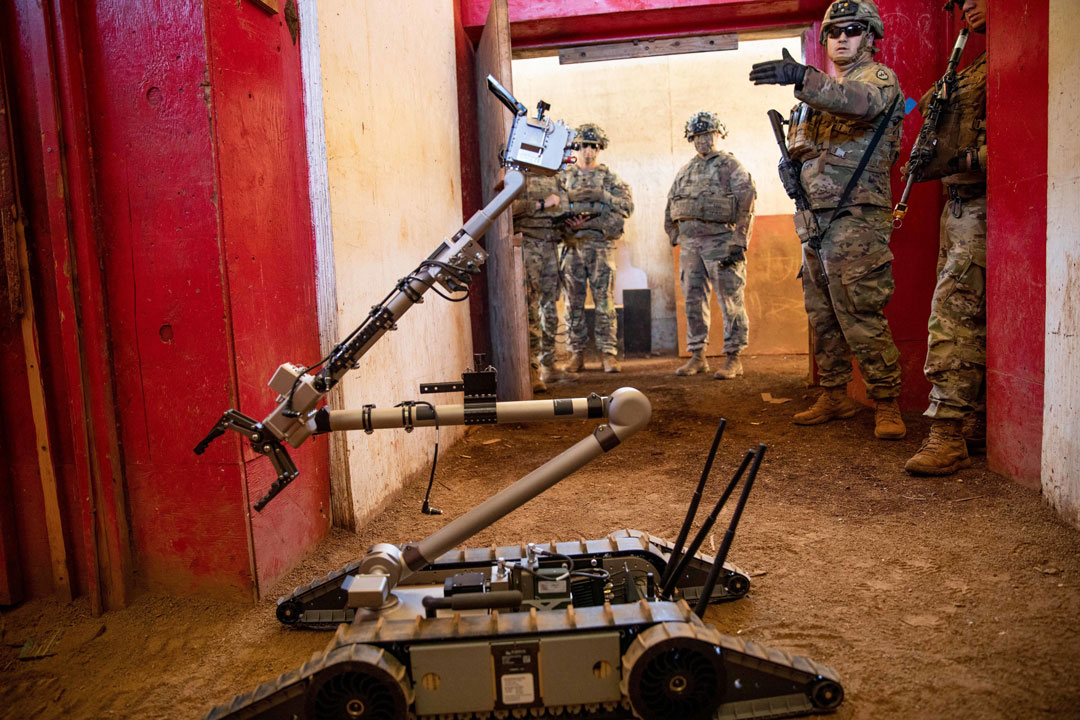
WHAT DID YOU SAY?: Noise levels differentiate the results of battlefield technology from commercial equivalents. Better microphones, custom noise filters, denoisification algorithms and automatic speech recognition models trained on noisy data may help improve results of voice control in the field.
(Photo by 1st Lt. Angelo Mejia)
PERFECTING VOICE COMMAND
Automatic speech recognition has several advantages in a tactical environment. It enables us to multi-task with a “heads-up, hands-free” approach. Natural language speaking is also the most effective way humans have to share information quickly. We want to be able to work with our devices the way we work with each other. Finally, there’s minimal learning curve for speech interfaces.
Voice control employs a transactional form of communication—a default behavior in response to a specified command. For example, “turn left,” or “begin recording.” Because there is a limited number of commands and they follow a particular syntactical pattern, often following a specific wake phrase (e.g., “Alexa” or “Hey, Google”), this level of interaction is not technically difficult to achieve. However, there is a spectrum of capability, from this type of basic automation on one end to conversational AI on the other end.
NOW WE’RE TALKING Much work has been done in the field of natural language processing, but it’s important to note that written communication can be very different from spoken communication. Spoken communication is often less formal, because it can afford to be so. Take, for example, the following dialogue, which could take place between two humans or between a human and a robot interface. Initiator: “Get that thing over there.” There are a few things happening in this dialogue. The initial command, “get that thing over there,” is ambiguous. Neither what the thing is nor where are precisely defined. The following lines go back and forth rapidly to clarify the request. The initiator indicates a location by pointing and the other party identifies the object of interest by asking if it’s the blue one. One thing that speech allows for is a quick exchange. Had the original request been in writing, the initiator would have been aware that the request was vague without sufficient context and might have written something like “retrieve the blue box from the northeast corner.” Otherwise, the back-and-forth of the written messages would have taken too long. There’s also a question of economy of effort—a speaker wants to use as few words as possible, while the listener needs the words to be precise. Often, a speaker can also rely on additional channels of communication to augment the content—for example, intonation, pauses, volume, expression or gestures. The medium of our communication affects the content. It’s a form of code-switching, where a speaker changes how they speak based on the audience. We could teach our Soldiers to speak in a manner that is more readily parsed by a computer agent, effectively forcing them to code switch, depending on whether they’re talking to man or machine. But let’s face it—if they take our language, the robots have already won.
|
Leveraging work from the University of Southern California’s Institute for Creative Technologies—a university-affiliated research center established by the Army—the U.S. Army Combat Capabilities Development Command’s Army Research Laboratory developed the Joint Understanding and Dialogue Interface (JUDI) capability. With JUDI, Soldiers won’t have to learn the language of a remote unmanned vehicle—they could say something like “move forward” or “get closer,” where the context is implied. Rather than identifying requests as invalid (e.g., “I’m sorry, my responses are limited.”), JUDI can enable an autonomous system to seek clarification, including when changes in the environment may have impacted the original request.
Two features set apart a conversational AI system from mere voice control. The first is the ability to take turns speaking and listening and the second is memory. If a user asks a commercial virtual assistant for a restaurant’s hours, and then for its location, the restaurant must be specified in both requests, even if one is made just after the other. Each request is consumed independently of the user’s history—it’s like having a conversation with a goldfish. In our conversations with our fellow humans, we leave a lot out because we assume a common frame of reference built on shared experience.
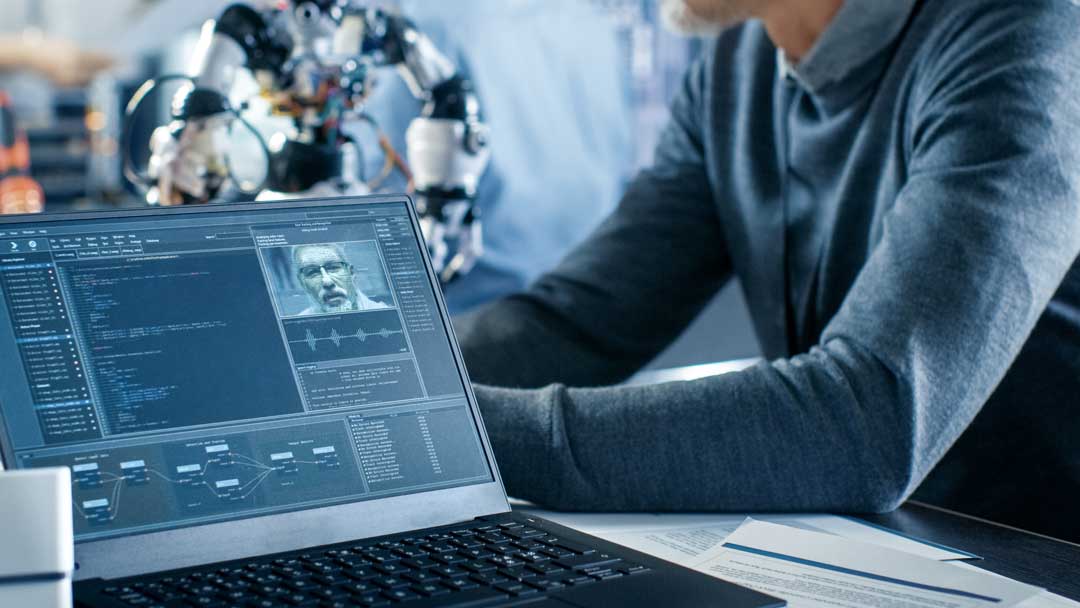
SAY IT AGAIN: The best, most effective way of communicating is through natural language speaking—getting AI agents to recognize natural language is key in future developments. (Image by Getty Images)
Memory is context of the past, but other forms of context are also useful, such as time and space, the task being performed, roles and the environment. For example, spatial context is important to an autonomous system to know where it can’t go (up a cliff) or shouldn’t go (off a cliff) and requires a specific sensor array to implement. A system should also understand a user’s specific preferences, style of speech, language and accent. The ability to recognize and adapt to a user, though more complex, means less training is required on the user’s part to adapt to the agent.
The third use case for automatic speech recognition, context analysis of voice communications, can be used for intelligence gathering, but also for maintaining situational awareness for our own forces—for example, detecting when Soldiers talking over the radio mention an enemy tank or incoming fire. Speaker detection is an important automatic speech recognition technique to build situational understanding—for example, being able to distinguish between participants in a conversation, a feature, called “speaker diarisation,” informs inferences about the relationship between the participants (i.e., different ranks and roles) and how that bears on the content of the discussion. For radio communications, speaker detection is also key to threading together conversations that may be coming through an operations center as a series of separate voice data packets.
The Army Rapid Capabilities and Critical Technologies Office (RCCTO) sponsors the Virtual Assistant for Mission Operations (VAMO) project. Working with the Massachusetts Institute of Technology Lincoln Labs, a federally funded research and development center, VAMO is exploring the use of text and speech interfaces to provide transcripts, autofill forms and summarize content. The VAMO team recently participated in a technical exchange with the Navy, which is working on a similar project, the Ambient Intelligence Speech Interface (AISI).
HEARING THROUGH THE NOISE
While we’re already starting to deploy automatic speech recognition-enabled systems, research challenges are still being addressed by our science and technology community to expand capabilities and improve outcomes. One of the most needed resources in this development is not expensive—it’s data. Machine learning approaches are promising, but require large quantities of data. Talk via radio, in command posts and in moving vehicles—both during routine operations and during engagements—are necessary to ensure that the models are both sensitive to changes like noise levels or phase shifts, and also robust enough to handle natural variety.
Noise is one of the main factors that differentiates the results of battlefield tech from its commercial equivalents. It’s also not likely that a single solution will fix this problem. Better microphones may help, as will custom noise filters, denoisification algorithms and automatic speech recognition models trained on noisy data. Not all noise is bad, either—markers such as disfluency (e.g., um, uh), pauses and stutters themselves provide information that is useful to understand the message, identify the speaker and even provide information about that speaker’s state of mind.
We must also be attuned not only to the benefits of automatic speech recognition, but also to the potential impact on Soldier performance. There’s almost always a story in the news about privacy fears and so-called “always listening devices.” Whether we’re talking about tapping into text chat or radio chatter, this is undoubtedly more intrusive than Google mining email keywords for ads because the output has a wider audience than the user. Because of this, deploying automatic speech recognition has the potential to change how our Soldiers communicate, or which media they use, even if we don’t specifically teach them how to talk like a computer.
Studies have shown that drivers engaged in conversation have a significantly slower response time than those who are not—and further studies have shown response time is even worse when the conversation partner is not a passenger, who might mitigate the response through their own awareness, but someone on the other end of a phone call. This certainly has implications for attention and focus on tasks; for example, when a Soldier is performing a demanding task like operating a vehicle and that Soldier must also speak an unrelated command at the same time, like reporting an observation of an enemy plane.
CONCLUSION
Over the past fifty years, there have been several “AI winters” when disappointment about the outcomes of AI investments led to periods of reduced interest and funding. Automatic speech recognition is not immune to this fate. As the acquisition community, we want to provide the best tools available. At the same time, if we field automatic speech recognition that performs below commercial expectations, trust becomes a factor in whether or not those tools are used. While the commercial world will no doubt continue development of this technology as long as there’s money in it, only some features of a tactical application are considered dual-use. Speaker detection, for example, has a use when more than one person shares a virtual assistant, but mitigating a tactical noise profile is less applicable to commercial devices. While several organizations, including the Joint Artificial Intelligence Center and the RCCTO, have started testing various open-source automatic speech recognition models for particular tasks, the initial results have shown room for improvement with a tactical noise profile. Training automatic speech recognition for high and variable noise levels is not something industry will prioritize for dual-use technology.
The stakes for technology reliability are higher in a tactical situation, where a mistake could result in the loss of a life rather than the wrong song being played. When we have a fight on our hands, it should be with the enemy, not with our technology, and we shouldn’t have to raise our voices just to be heard.
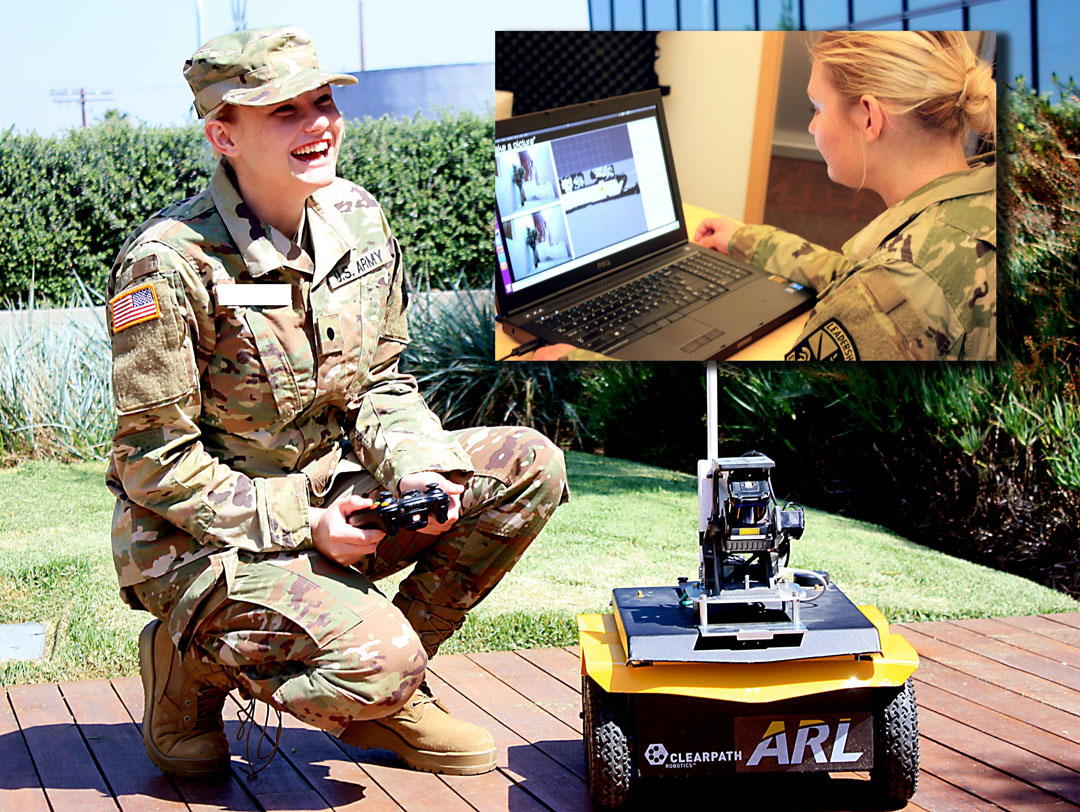
EASE OF USE: With JUDI, Soldiers will be able to say simple commands like “move forward” where the context is implied, and the system can seek clarification. (Image by U.S. Army)
For more information, contact Thom Hawkins at jeffrey.t.hawkins10.civ@mail.mil. For more information about RCCTO and VAMO, contact Sean Dempsey at sean.e.dempsey.ctr@mail.mil and for more on JUDI, contact Matt Marge at matthew.r.marge.civ@mail.mil.
THOM HAWKINS is a project officer for artificial intelligence and data strategy with Project Manager Mission Command, assigned to the Program Executive Office for Command, Control and Communications – Tactical, Aberdeen Proving Ground, Maryland. He holds an M.S. in library and information science from Drexel University and a B.A. in English from Washington College. He is Level III certified in program management and Level II certified in financial management, and is a member of the Army Acquisition Corps. He is an Army-certified Lean Six Sigma master black belt and holds Project Management Professional and Risk Management Professional credentials from the Project Management Institute.
DR. REGINALD HOBBS is chief of the Content Understanding Branch at the U.S. Army Combat Capabilities Development Command Army Research Laboratory. He earned a Ph.D. and an M.S., both in computer science, from the Georgia Institute of Technology and a B.S. in electronics from Chapman University. He has research interests and experience in the areas of software engineering, network science, natural language processing, artificial intelligence and cognitive science. He serves as an adjunct professor on the faculties of Howard University and the University of the District of Columbia.