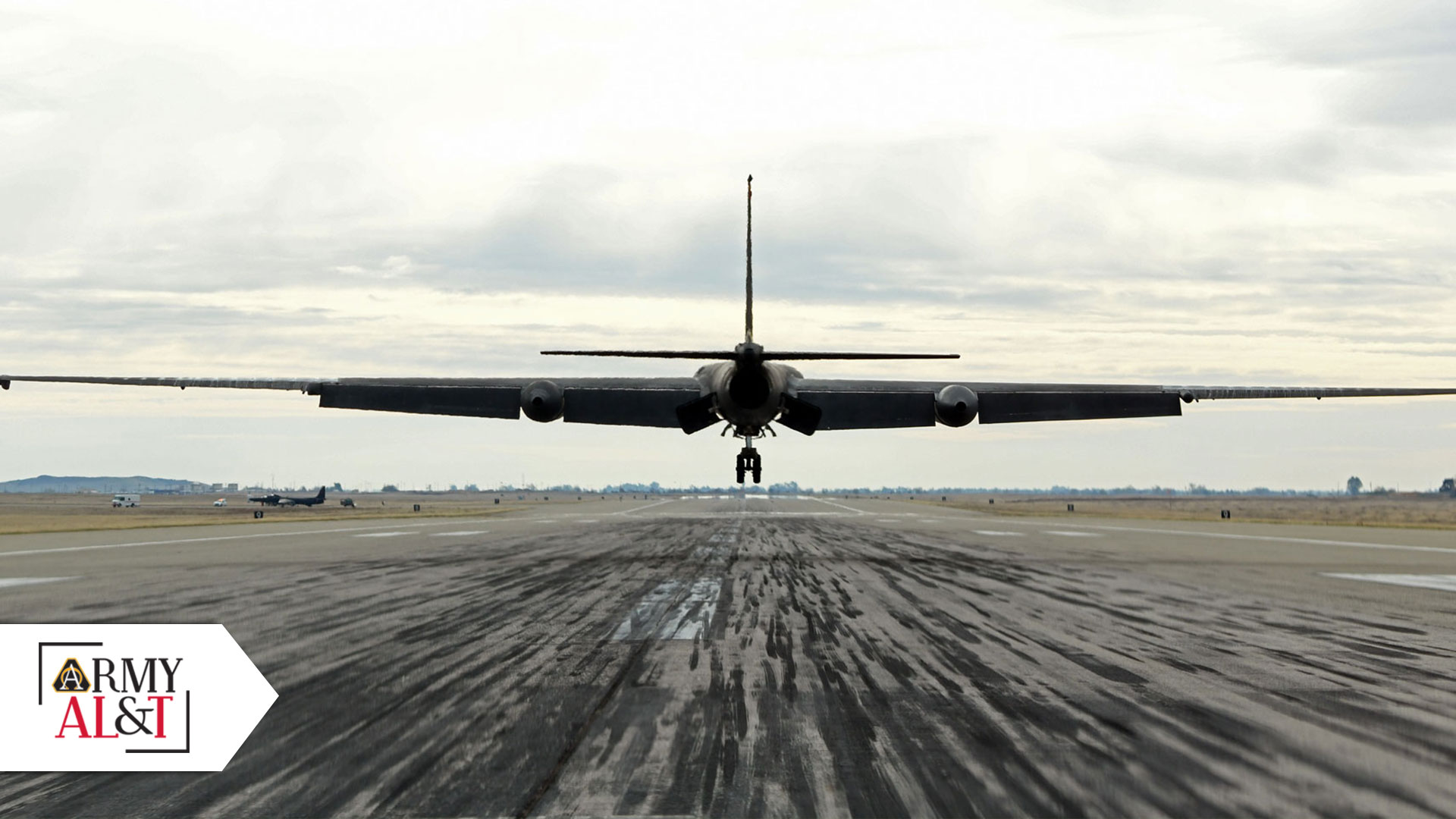
FLYING DRAGON: A U-2 Dragon Lady assigned to the 9th Reconnaissance Wing prepares to land at Beale Air Force Base, California, on Dec. 15, 2020. This flight marks a major leap forward for national defense as artificial intelligence took flight aboard a military aircraft for the first time in DOD history. The AI algorithm, developed by Air Combat Command’s U-2 Federal Laboratory, trained the AI to execute specific in-flight tasks that would otherwise be done by the pilot. The flight was part of a specifically constructed scenario pitting the AI against another dynamic computer algorithm in order to prove both the new technology capability, and its ability to work in coordination with a human. (Photo by Airman 1st Class Luis A. Ruiz-Vazquez, U.S. Air Force)
PEO C3T’s pathfinder project will pave the way for future AI enablers.
by Thom Hawkins and Peter Schwartz
There are times when our ignorance is unquantifiable, and that can put our plans at risk. As former Secretary of Defense Donald Rumsfeld said, “There are known knowns. There are things we know we know. We also know there are known unknowns. That is to say, we know there are some things we do not know. But there are also unknown unknowns, the ones we don’t know we don’t know.” The best way to reduce the risk of “unknowns unknowns” is by exploring the domain in a purposeful way. You’re sure to encounter both the expected and the unexpected, and along the way, leave in place a path for others to follow. It’s only by facing those unknowns head-on and finding a path through them that you can make your vision a reality and not just a castle in the sky.
As the materiel developer for the tactical network and the mission command and fires control applications that run on the network, the Program Executive Office for Command, Control, Communications – Tactical (PEO C3T) has a particular niche to scratch when it comes to artificial intelligence (AI). For example, most out-of-the-box AI solutions assume seamless connectivity between data input, data analysis and model output to point of use. In a tactical environment, we must compensate for denied, degraded, intermittent or limited connectivity—including low-bandwidth transmission, the need for applications to operate with variable levels of processing power, and being able to hold data and prioritize transmission when connectivity is available. We’re unlikely to find a single solution to meet our needs as well as operate under our constraints; if we do, it will likely be unaffordable. To solve this problem, PEO C3T has begun a brand new pathfinder project to tackle identified gaps like infrastructure and data availability to build a foundation for enabling AI across our portfolio in blocks of capability.
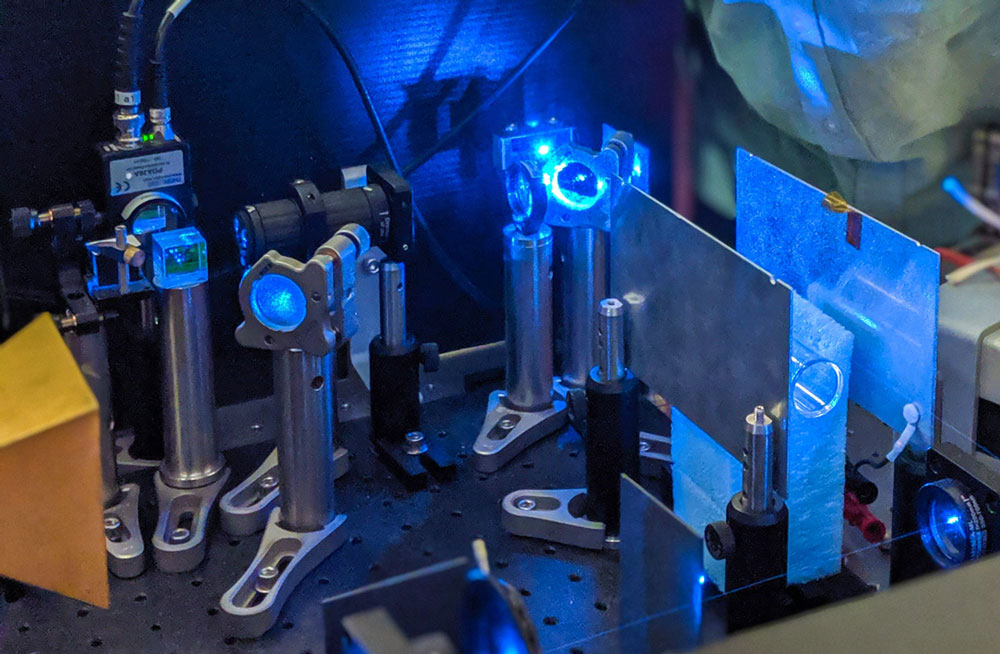
QUANTUM LEAPS: Researchers from the U.S. Army Combat Capabilities Development Command’s Army Research Laboratory and Tulane University combined machine learning with quantum information science, or QIS, using photon measurements to reconstruct the quantum state of an unknown system. The Army hopes to harness artificial intelligence and machine learning to advance its effectiveness in tactical environments. (Photo courtesy of the U.S. Army Capabilities and Development Command (DEVCOM) Army Research Laboratory)
UNMAGICAL REALITY
There’s a running joke that artificial intelligence (AI) works like magic. You take all of your problems—inconsistent or nonexistent data, or even an overwhelming amount of data, unpredictable circumstances, the advancing speed of threats—you stuff them into a cauldron, sprinkle in some AI and the potion turns to gold—and it tastes great, too! We know it works because we’ve seen the demonstrations—sanitized data, locked in a castle-like data center, surrounded by a moat of clear distinction.
The reality is that there is no monolithic data castle and we need more than magic. Tactically, a castle is great for defending your home turf, but not such a great utility for an expeditionary force. In our rush to adopt AI, we defaulted to the acquisition model we all know—identify explicit requirements for AI, pass them off to a systems contractor, field the result and start fresh with the next set of requirements. We haven’t thought much about what’s different with AI, because we can’t afford the luxury of time when we’re in competition with nations less concerned about the ethics and implications of deploying this advanced technology.

FIGURE 1: PEO C3T’s potential design pattern for an AI reference architecture. (Image courtesy of PEO C3T)
In the early days of 2020, before the year was defined by the COVID-19 pandemic, PEO C3T convened the first of several AI-focused workshops to survey stakeholders—including requirements developers, testers, science and technology—to identify potential use cases for AI, as well as what the roadblocks would be to deploying AI in the field. The results of that workshop, further refined over the ensuing months, demonstrated that there was a lot missing from the bigger picture—like access to operational data and a lack of common infrastructure—to make AI effective and sustainable.
OVERCOMING ROADBLOCKS
The way to best approach these AI roadblocks, PEO C3T determined, was engagement through an AI pathfinder project. The project will spin out a series of AI-enabled capabilities that were carefully selected to force the PEO and its partners to work through the barriers discovered during the workshops. For example, we’re developing applications that will be able to identify key information from an operational order and feed it automatically into a range of systems, but we’ve only been able to gain access to four examples of operational orders, and most of them are from the training environment, which may not fully represent conditions in the tactical environment. We need data-sharing agreements that span Army or DOD tactical operations. Currently, orders, communications and other types of content are owned by the combatant commands, and are thus classified with a need to know, limiting access for research and development. In addition to providing an opportunity to investigate, document and bridge these gaps, the pathfinder project will partner across Army organizations, leveraging ongoing efforts, to produce useful AI-enabled operational capabilities for Soldiers and leave behind new or enhanced development processes and infrastructure for future AI projects.
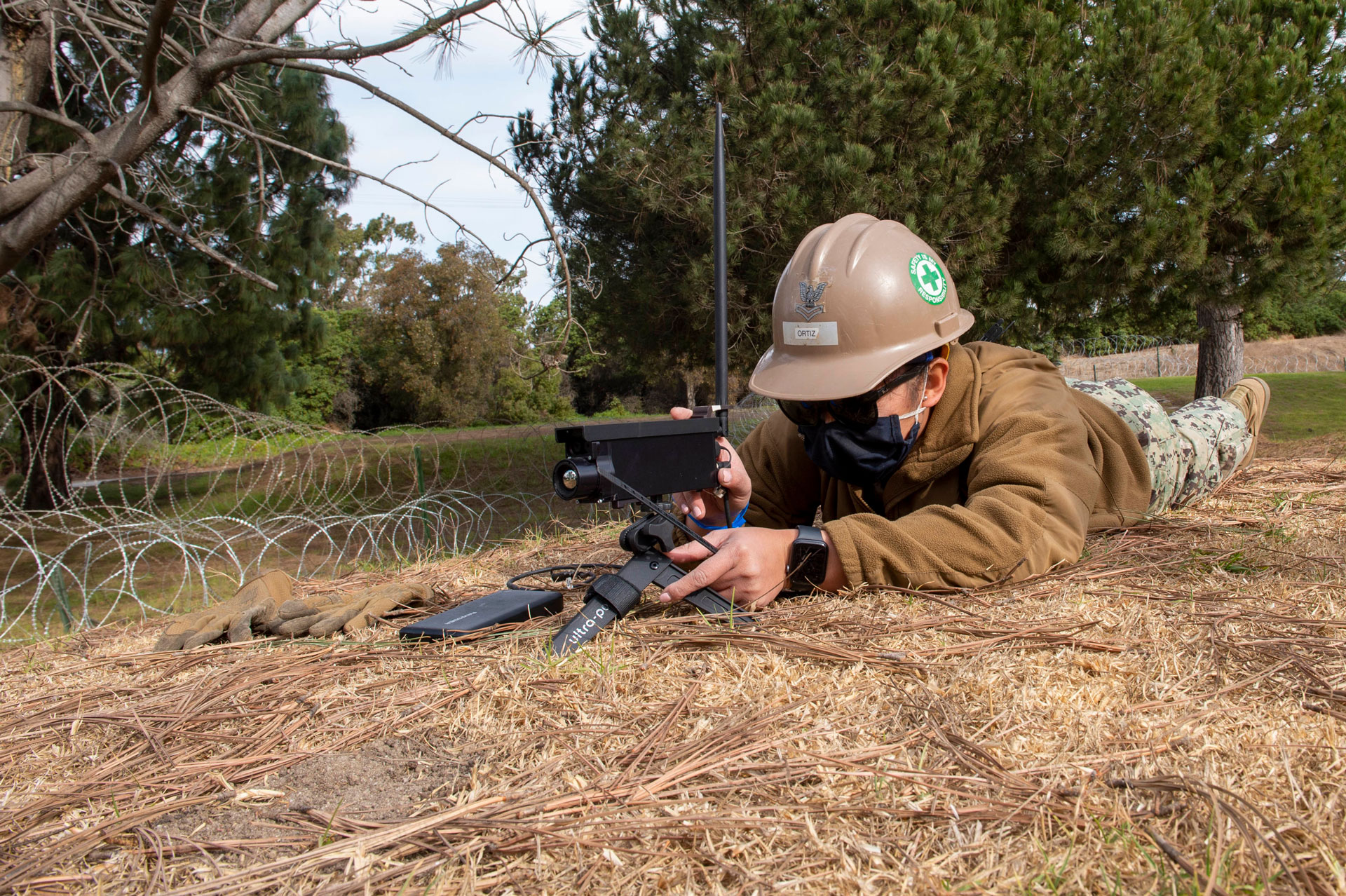
INTELLIGENT SURVEILLANCE: Construction Electrician 2nd Class MaryJoy Ortiz, with Naval Mobile Construction Battalion 5, sets up a Gantz-Mountain Surveillance camera Dec. 7, 2020 at Naval Base Ventura County, California. The battalion augmented ground surveillance with the revolutionary tactical surveillance system, using artificial intelligence and behavior analysis at-the-tactical edge, throughout its second Command Post Exercise. (Photo by Mass Communication Specialist 1st Class Stephane Belcher, U.S. Navy)
The PEO C3T AI pathfinder project takes a risk management approach to implementing AI infrastructure and functions in mission command applications, acknowledging the uncertainty in acquiring these capabilities in an acquisition system that was designed for tanks and bullets, and working with partners across the domain to reduce that uncertainty through targeted AI development:
- The first phase, for the 2021 fiscal year, is to develop the ability to complete a medical evacuation (MEDEVAC) request from a chat window. The application will identify relevant information from a chat in progress and use it to generate a nine-line MEDEVAC call, allowing the user to review and send the call, rather than having to manually enter the information in a separate window. Even this relatively modest undertaking already requires access to training data and a limited ontology.
- The second phase will add situational awareness across text chat and voice communications, providing near real-time alerts from the massive amounts of tactical communications traffic, based not only on keywords, but also on related topics. This may allow a commander to get an alert when a specific location or target is mentioned, or identify trends such as heightened traffic in a particular location that may warrant attention.
- Future phases will add layers of complexity with mission validation reasoners and decision tools to optimize courses of action.
The pathfinder project will reexamine the acquisition process to determine the flexibility and infrastructure needed to enable AI across multiple systems. System requirements are written and executed narrowly, according to prescribed funding and schedule, and dependencies on undeveloped resources like a knowledge base or ontology represent risk. However, this is a chicken and egg problem, because no one will build the common resources needed without the funds allotted through acquisition. Just as a smartphone application can be given permissions to use your phone’s existing camera, microphone or date and time provided by the hardware and operating system without redundancy, an AI application that provides reasoning for, say, the ability to automatically validate mission requirements, should be able to make use of a common knowledge base, existing reasoners, or data from information extractors. As we move toward a more efficient AI, a more general AI that makes use of common resources, we need to support through acquisition an architecture that will allow plug‑and-play upgrades and enhance competition in a rapidly evolving industry.
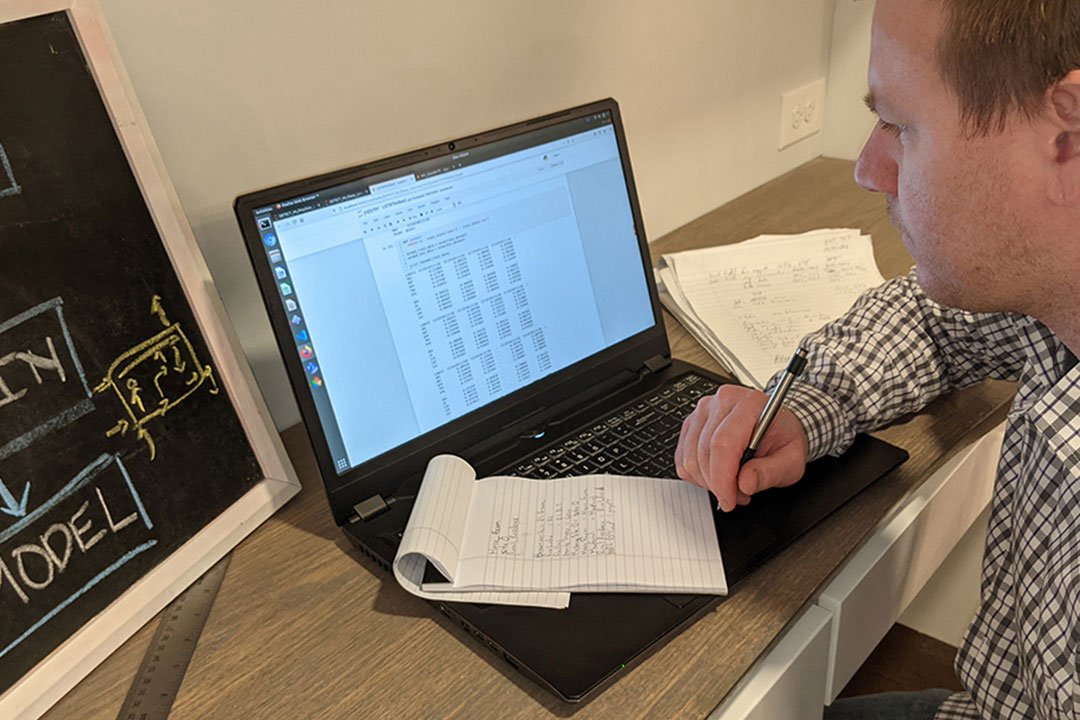
THE AI STEWARD: Patrick Riley, who is leading the DEVCOM Chemical Biological Center’s artificial intelligence and machine learning initiative known as STEWARD, coding a software program that uses artificial intelligence and machine learning to advance his research. Artificial intelligence and machine learning is a next-generation computer capability that holds the potential for changing everything from how people live and work to how wars are fought and won. (Photo by Jack Bunja, U.S. Army Combat Capabilities Development Command Chemical Biological Center)
A COMMON ONTOLOGY
As the basis for an AI reference architecture, PEO C3T is considering a design pattern (see Figure 1) to reduce stovepiped elements and rely on a shared data source that will alleviate data flow bottlenecks in an environment with denied, degraded, intermittent or limited connectivity. An information extractor pulls in data from an external system into a shared data resource, or knowledge base. The knowledge base stores data, including both static data like system performance specifications that don’t change, and dynamic data like weather or the safety of a given route. Each reasoner is an algorithm that makes logical inferences about data; it reads data from the knowledge base, processes it and then pushes it back into the knowledge base where it can be consumed by any other reasoner. Finally, an information encoder makes data available in any format required by an external system. A “data fabric” may be used to connect the data of all systems in the portfolio, potentially providing the information extraction and encoding features.
The common data model that these software components share can be expressed as an ontology—a semantic model of data that describes how the data are related to one another. The ontology provides a unified basis for reasoners, so they no longer require specialized point-to-point data translators. For example, if a squad is required to travel 100 kilometers to its next destination in a vehicle that has a fuel economy of 10 kilometers per gallon, but the squad only has eight gallons of fuel remaining, a reasoner might identify that the squad does not have sufficient fuel to complete its mission. One vendor could develop a reasoner to validate mission resources and push the results into the knowledge base, and another vendor could read in that data to support course-of-action analysis. By pushing data into a common data store and organizing it according to a common ontology, the information is always available to immediately support any new concept that a developer can dream up—without requiring months of additional software integration. While many applications will need this to be available, there is no precedent for building and maintaining an ontology that is used by more than one system—it’s not in anyone’s mission, nor in their funding, but it will be absolutely necessary to sustain anything beyond a very narrow AI in the field.
CONCLUSION
Each phase of the pathfinder project leaves behind infrastructure and development tools that will help the following phases and future AI enablers. If successful, the PEO C3T AI pathfinder will significantly reduce the time it takes to develop a new idea from concept to deployment, allowing PEO C3T to be much more responsive to the emerging needs of Soldiers.
There’s no magic here. This quest is about hard work and collaboration. We’re partnered across the enterprise, with Army Futures Command for requirements development and science and technology; the Rapid Capabilities and Critical Technologies Office; and federally funded research and development centers. Each partner brings a different perspective and a different part of the solution. We’ve left behind the castles in the sky and are maneuvering across the tactical landscape, knowing that we can use AI to adapt to new challenges.
For more information, contact Thom Hawkins at jeffrey.t.hawkins10.civ@mail.mil or visit the website for PEO C3T at https://peoc3t.army.mil, or the Project Manager for Mission Command at https://peoc3t.army.mil/mc.
THOM HAWKINS is the project officer for AI and data strategy with Project Manager for Mission Command, assigned to PEO C3T at Aberdeen Proving Ground, Maryland. He holds an M.S. in library and information science from Drexel University and a B.A. in English from Washington College. He is Level III certified in program management and Level II certified in financial management, and is a member of the Army Acquisition Corps. He is an Army-certified Lean Six Sigma master black belt and holds Project Management Professional and Risk Management Professional credentials from the Project Management Institute.
PETER SCHWARTZ is a principal AI engineer for MITRE Corp. in the Artificial Intelligence & Autonomous Systems department where he serves as the Artificial Intelligence Joint & Services Domain subject matter expert. He supports multiple DOD sponsors as an AI subject matter expert and leads MITRE’s Army AI Community of Practice. He has taught AI for the MITRE Institute and has served on a variety of AI panels and conference committees. Before joining MITRE in 2016, he was a senior data scientist at Penn Medicine and previously a senior mathematician at ORSA Corp. He received a Ph.D. in computer science and intelligent systems from the University of Michigan in 2007, and a B.S. in computer science and a B.A. in psychology from the University of Maryland in 2001.
Read the full article in the Spring 2021 issue of Army AL&T magazine.
Subscribe to Army AL&T – the premier source of Army acquisition news and information.